Unlocking the Potential: Agriculture Datasets for Machine Learning
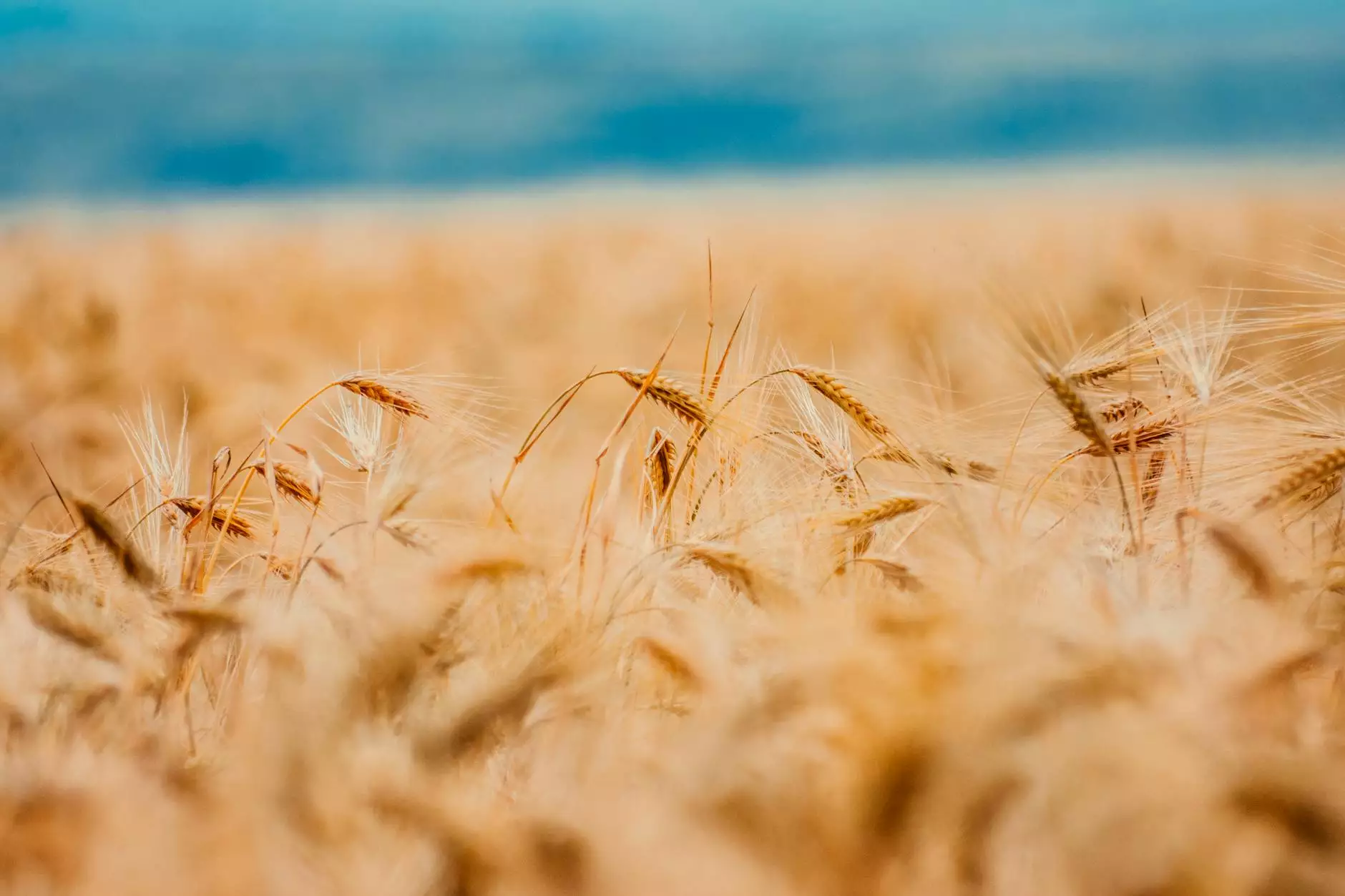
Introduction to Agriculture and Technology
The intersection of agriculture and technology is reshaping the way businesses operate. Traditional farming methods are increasingly being complemented by advanced technologies such as machine learning and data analytics. One of the key components fueling this transformation is the availability of extensive agriculture datasets for machine learning.
Understanding Agriculture Datasets
Agriculture datasets encompass a wide range of data collected from various sources within the agricultural sector. These datasets may include:
- Crop yield data
- Soil health statistics
- Weather patterns
- Pest and disease reports
- Agronomic research data
This data plays a crucial role in helping businesses make informed decisions, optimize resources, and mitigate risks associated with farming operations.
The Role of Machine Learning in Agriculture
Machine learning is a subset of artificial intelligence that enables systems to learn from data and improve over time without being explicitly programmed. In the context of agriculture, machine learning algorithms can analyze vast amounts of data to identify patterns and predictive insights. For instance:
- Pest Prediction: By analyzing historical pest data and current environmental conditions, farmers can anticipate pest outbreaks and take preventive actions.
- Yield Forecasting: Machine learning models can predict crop yields based on parameters such as weather forecasts, soil conditions, and crop management practices.
- Resource Optimization: Data-driven insights can help optimize the use of water, fertilizers, and pesticides, enhancing sustainability.
Benefits of Using Agriculture Datasets for Machine Learning
The application of agriculture datasets for machine learning offers a multitude of benefits that can significantly enhance business operations in the agricultural sector:
1. Improved Decision Making
By leveraging machine learning algorithms, farmers and agribusinesses can make data-driven decisions that lead to higher productivity and profitability. For instance, optimizing planting schedules based on climate data can lead to better yields.
2. Enhanced Efficiency
Automating processes such as irrigation and fertilizer application not only saves time but also reduces costs. Machine learning models can analyze data in real-time, adjusting operations for maximum efficiency.
3. Predictive Analytics
Predictive analytics powered by agriculture datasets for machine learning helps farmers foresee issues before they arise, enabling proactive management strategies that safeguard against losses.
4. Sustainable Practices
Data analytics supports sustainable farming practices by promoting efficient resource use, reduced chemical input, and improved land management, aligning with the global movement towards sustainable agriculture.
Case Studies: Successful Implementations
Several businesses have successfully integrated agriculture datasets for machine learning into their operations:
Example 1: Precision Agriculture
A precision agriculture startup utilized machine learning algorithms to analyze satellite imagery and soil data. By predicting the optimal planting times and inputs, the company increased crop yield by 30% compared to traditional methods.
Example 2: Crop Disease Management
A large agricultural corporation implemented a machine learning model to identify symptoms of crop diseases using image recognition technology. This approach allowed them to implement targeted treatment strategies, reducing crop loss by half.
Challenges in Using Agriculture Datasets for Machine Learning
While the potential of utilizing agriculture datasets for machine learning is vast, there are challenges that businesses must navigate:
- Data Quality: Inaccurate or incomplete data can lead to poor model performance. Ensuring high-quality data is essential.
- Integration: Merging datasets from different sources can be complex and requires robust data management practices.
- Skill Gaps: There is often a shortage of skilled professionals who can analyze data and build machine learning models.
Future Trends in Agriculture and Machine Learning
The landscape of agriculture is continuously evolving. Here are some trends to watch as the industry embraces machine learning:
- Increased Data Availability: With the advent of IoT devices, farmers will have access to real-time data that can feed into machine learning models.
- Automation in Farming: Drones and autonomous machines will increasingly rely on machine learning to operate efficiently.
- Focus on Sustainability: The demand for sustainable farming practices will drive the development of machine learning solutions that promote eco-friendly farming.
Conclusion
The integration of agriculture datasets for machine learning is a game-changer for businesses in the agricultural sector. By harnessing the power of data, companies can make informed decisions, optimize their operations, and contribute to the sustainability of our food systems. As advancements in technology continue to unfold, the potential for innovation in agriculture is limitless. Embracing these changes not only enhances productivity but also paves the way for a brighter, more sustainable future in farming.
For more insights on how to leverage machine learning in agriculture and to enhance your business operations, visit Keymakr.com.
agriculture dataset for machine learning